AI/ML in Investment and Risk Management: Recent Applications, Use Cases, and Implementation Challenges
By Ai4February 27, 2020
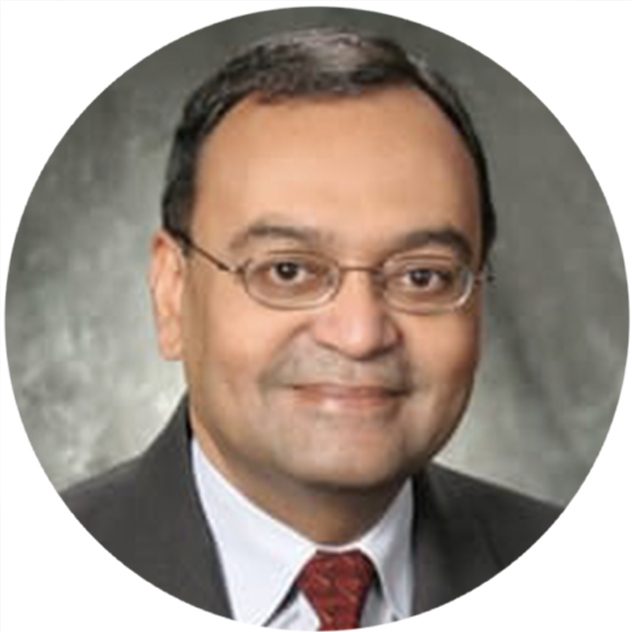
Managing Director - Head of
Global & Macro
PGIM Fixed Income
Introduction
Investing is a completely different ballgame from many of the other applications where AI/ML has been spectacularly successful. It presents some unique challenges both to the data scientist and the portfolio manager.
Here, Arvind discusses some of the most promising techniques, including natural language processing, regime classification, and pattern recognition, showing how they can be applied to investment-related use cases.
Biography
Arvind Rajan, PhD, is a managing director and head of global and macro at PGIM Fixed Income, heading the FX, Global Bond, Emerging Markets Debt, Investment Strategy and Economic Research teams.
Challenge: looking for solutions that can give customers an edge in investing.
Once you identify what’s useful, then you can actually try to apply it to asset management and risk management. It’s often difficult to implement new technology in an organization that’s used to doing things in a different way. The asset management industry and banking industries are hundreds of years old. Integrating quantitative techniques and data to traditional businesses is a major challenge.
What customer data is most relevant (and what isn’t)?
We collect 1 billion pieces of data about each human each day. Yet are there even ten useful things per person each day worth knowing? Most of this data is noise, so we use AI to find the relevant bits. However, there can be tremendous inefficiencies if not guided properly.
What can big data and analytics tell us that no one else can?
Any new value-added information gleaned by investors from alternative data sources:
- Must not (yet) be available from existing sources (banks, brokers)
- Must not (yet) be disclosed by the management of the issuing entity (country or corporation)
- Must not (yet) be incorporated into the market price
Conclusion: the majority of new information is likely to be short-lived, of limited individual importance and/or hard to extract.
Corporations may withhold information from their investors for a number of good reasons:
- They do not want the competition to know about it.
- The information may have the effect of transferring value between stocks and bonds.
- The information is not due to be revealed yet.
- Management is not aligned with other stakeholders
- The information may pertain to what other investors think or are doing.
- The information may be unknown to both management and to other market participants.
Conclusion: AI/ML and Big Data initiatives try to ferret out and exploit these hidden elements.
Recent applications (and successes) in asset management
Alpha generation
Alternative data: identify tactical trading opportunities with more timely information than traditional data sources; more robust modeling of performance drivers at company level.
Incorporating AI/ML to alpha generation requires new skills and processes. You’ll need data engineers, data scientists, quantitative analysts, and professionals with domain knowledge.
Natural language processing: analysis of transcripts, anomaly detection of SEC filings; prioritization of news flow; identifying trending themes across sectors. There are a number of potential uses for asset managers, including: “scoring” text-based reports by their potential impact on a security, identifying “new” news, and prioritizing news flow for analysts.
Classification and clustering algorithms: regime classification, identify similarity patterns for prior fundamentals at company level.
Risk Management
Cluster analysis with machine learning: identify groups of bonds that behave similarly in thin markets to predict bond liquidity characteristics
Sentiment analysis using natural language processing: identifying tweets regarding major banks under supervision to predict changes in risks to the banking system by predicting changes to financial ratios and market levels.
Use case: Bank d’ Italia used sentiment analysis of tweets with unsupervised methods to predict risks to major Italian banks.
Pattern recognition with unsupervised learning: model validation for regulatory or trading purposes: identify a small anomalous fraction of simulation runs of projected scenarios generated by a stress testing model that are invalid due to data input or other reasons (these can be re-run).
Other applications
Manager selection: data-driven approaches to better assess manager performance. Is the manager meeting certain benchmarks?
Trading/execution: large data sets of intra-day activity can help refine trading approaches to optimize execution; street firms using NLP to scrub all trade inquiries regardless of source.
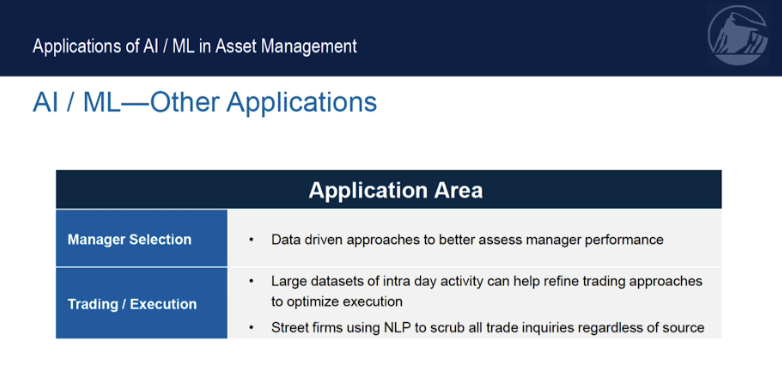
Key challenges in Integrating AI
- AI techniques transpose human processes; what is hard becomes easy (example: large-scale computations) while what is easy may be hard (example: a leaky faucet).
- Enormous amounts of new data, most of which is noise.
- Standalone AI is easier to implement but its scope is often limited.
- Augmentation with AI is more broadly applicable but harder to incorporate.
- The field of investment management presents special challenges because of the large role of game theory and human behavior in financial markets.
Implementation Changes
Challenges in modifying staff and process:
- Staff skill shortages: fundamental manager staff may lack analytics/data science staff skill sets
- Investment process incompatibility: may need major changes to incorporate data and AI/ML techniques
- Cultural resistance: changes may encounter reluctance/resistance from fundamental research staff and portfolio managers.
Value hard to prove: value added is hard to quantify when the investment process is partly subjective.
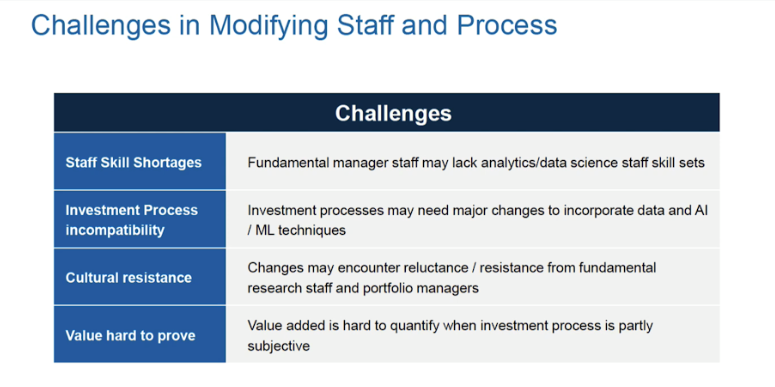
Challenges in using alternative data
- Uncertain value in using alternative data: value is uncertain and dissipates quickly; signal may already be incorporated; difficult to isolate/measure incremental value. You don’t really know how much value is added by any new set of data, and you don’t know how long it will last.
- Data availability, quality and quantity: firm’s ability to suitably organize their internal data is limited; large data sets, particularly news and sentiment come from disparate sources and include substantial noise; most data sets have limited history, making it difficult to test through various market environments.
- Speed of change: AI/ML is constantly evolving -- expensive to keep up, particularly given uncertain value. Continuous process requiring constant evaluation of best data sets and algorithms.
Challenges using machine learning techniques
- Overfitting of models: given larger number of inputs, enormous computing power, and automation of data science platforms, we need to be cautious not to overfit data.
- Sampling period selection: out-of-sample tests result in selecting models that work well for a specified out-of-sample period. There may be a bias to overfit the “out-of-sample” period.
- Econometric time series data issues: economic data comes late, with lags and restatements.
- Black box models: many machine-learning models are non-linear and structurally opaque (example: black boxes), casting doubts on output stability, why they work and for how long.
- Ethical concerns: model selections may contain biases that contravene the law or company policy.
Recent Posts
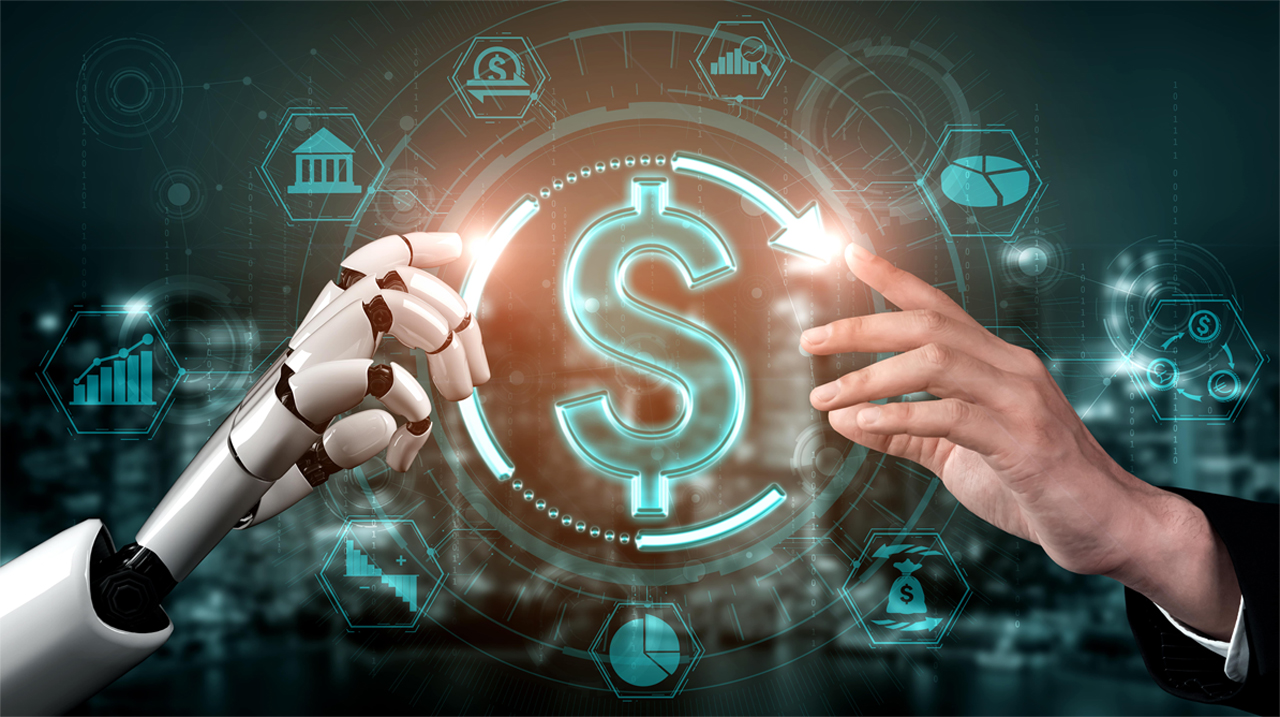
5 Effective Risk Management Strategies When Trading in Crypto
Cryptocurrency has slowly made its way into the mainstream, and more people have begun thinking...
By Trix MejiaFebruary 25, 2022
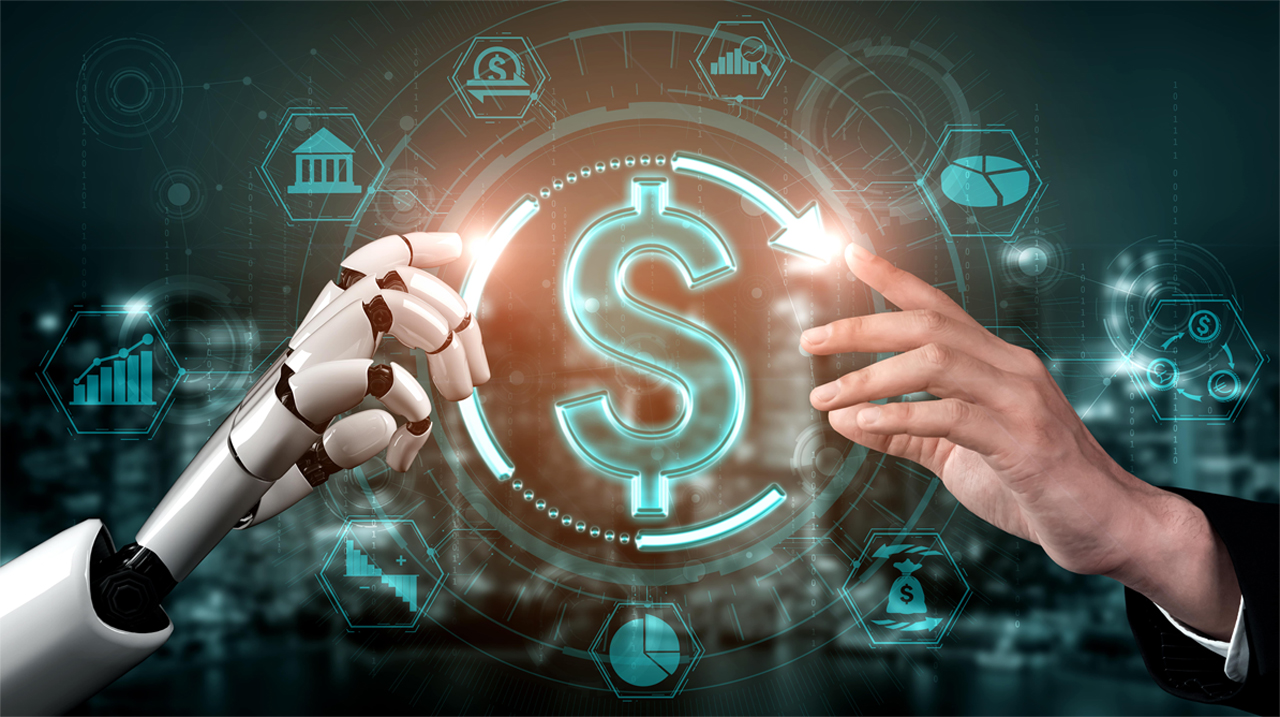
How AI Will Be Impacted By the Rise of NFTs?
In 2021, there was no way to really escape the online chatter about NFTs. In...
By Ellen KaneFebruary 15, 2022
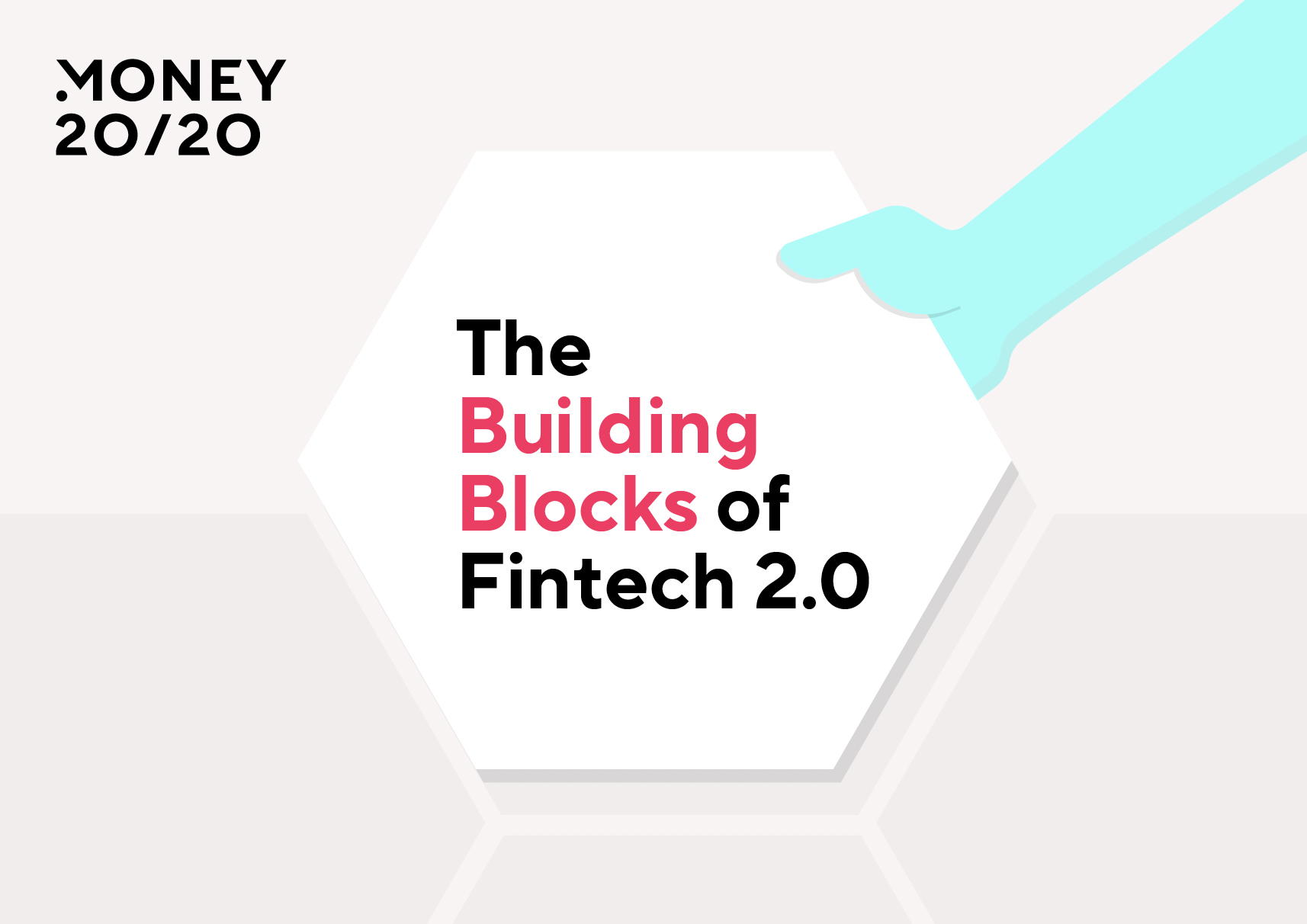
Money 20/20: The Building Blocks of Fintech 2.0
In this whitepaper we will take a look at the drivers setting the scene for...
By Sanjib KalitaAugust 11, 2021