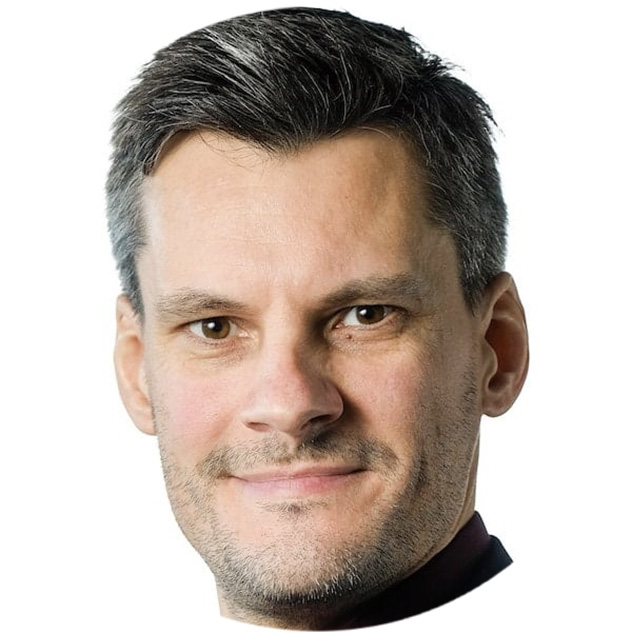
VP, Data Science & AI
Astrazeneca R&D
Intro to AstraZeneca R&D
Here we see an end-to-end case study of how AI and ML come together in a single company. AstraZeneca is a global, researched-based pharmaceutical organization located in the U.K., with offices located close to science clusters: Gaithersburg, MD, Gothenburg, Sweden and Cambridge, England.
The company is very innovation driven and led by science -- determinedly research and development (R&D)-centric. An enormous amount of money is invested in R&D, along with a large pipeline of medicines that are constantly being discovered and developed.
AstraZeneca focuses on three main therapy areas across key platforms:
- Oncology
- Cardiovascular, renal and metabolism
- Respiratory
AstraZeneca’s approach to data science and AI
The goal is to better understand diseases, discover drugs, and put those drugs through the necessary tests and trials. Ultimately, the hope is to get the drugs approved and to the patients who so desperately need them.
Jim notices that R&D is increasingly generating more (and different types of) data at every point along the process -- not just company-generated data, but all data being received from various sources in the outside world. All of this data can contribute to specific tasks in this chain.
How do you achieve mastery of this data and get the most out of it? The second thought: what are we trying to do with all this data?
The goal: achieving data type intersection
Over time, Jim’s department wants to get better at creating intersections between data types. As a result, new insights, hypotheses and science may be gained. Ultimately, machines should reason over vast quantities of interconnected data that may actually suggest useable hypotheses. The goal is to help human scientists do their job more effectively.
Note: people, not machines
Important point stressed: AstraZeneca does not want technology to replace the human scientist. Instead, technology should be only a digital assistant to the human scientist. The company employs a single group that thinks about an entire problem space from end to end.
However, large pharma companies will quickly fall behind if AI is not adapted. AstraZeneca is looking at AI to be a way to improve the way scientists work, not to replace them.
Using data to develop insight
What can we do with all this data? It can be used to discover deeper and more sophisticated scientific insights in patients, medicines and diseases.
AI can be applied to the following goals and subject areas, among others:
- Genomics
- Disease understanding
- Drug design and synthesis
- Personalized medicine
- Imaging
- Clinical
AstraZeneca’s current AI status
The company strives to move faster in order to pick the “winners” more effectively. Here’s how a win is defined: speeding up the time to develop a medicine (using a closed-loop process) and getting that medicine to the patients who need it. Probabilities of success can then be more predictive and born out of reality.
For example, when a medicine is put to trial, we can know ahead of time how it’s more likely to succeed.
To sum up, the immediate goals:
- Going faster
- Picking the winners
- Getting the medicine to patients more effectively.
AstraZeneca’s framework to drive innovation in data science and AI
- Control: developing standards, governances and policies, ensuring trust, privacy and security in data.
- Organize: processing, formatting, profiling, structuring, capturing, meaning in, and developing relationships between data.
- Insight
- Learning and development: investing in internal education to ensure everyone has the opportunity to take advantage of what data science and AI can do.
This AI plan is set up in a “hub-and-spoke” framework
Data science is a team sport, so the goal is to bring the teams together.
Most companies don’t want multiple data platforms and policies. Every department should have a clear purpose and sync seamlessly with other departments/teams.
Automating drug discovery will become better and faster with the help of AI
AstraZeneca found that AI that can speed along experimental projects in hours instead of weeks. Machine learning can analyze the results and automatically suggests tweaks.
The goal is to figure out how to fully automate this process, from the initial chemistry stage to creating the final drug product. The network knows what “good” looks like, and it keeps going back and trying different things to get to that “good.”
Chemists and researchers use machine learning to create a tool. For example, the AI neural network is working to find just the right design. There is huge potential there.
AI in imaging
We know that computers will eventually be able to perform imaging much better than it does now -- the benefits would be all across the board.
Algorithms can help us analyze images quickly, and more accurately cheaply. Human pathologists (not machines) will help interpret all of this information. It will save a lot of time instead of having to spend hours looking down a microscope and making informed decisions about which medicines we take forward.
The most effective way to utilize imaging is to create a closed feedback loop so that patients can better manage their condition in the future.
Potential & Pitfalls
Challenges:
- the effort required to make data findable, accessible, interpretable, and reusable (F.A.I.R.) is often downplayed.
- We need to manage the hype.
- Ethics of algorithms are still undetermined
Opportunities:
- We have access to more data than ever before.
- Make the most of the hype! This area is completely oversold. It’s being talked about so much.
- Spotlights on ethics drives better understanding.
What’s next
A look to the future:
- Joint research centers with academia
- Innovating with biotech
- Partnering with industry
The place where AI and data science impacts lives
- Improving our understanding of disease and uncovering new targets.
- Driving personalized medicine strategies
- Speeding the design and delivery of potential new medicines for patients.