The Ethics of AI: Who will be Responsible for the Decisions of AI Applications?
By Ayodele OdubelaApril 20, 2020
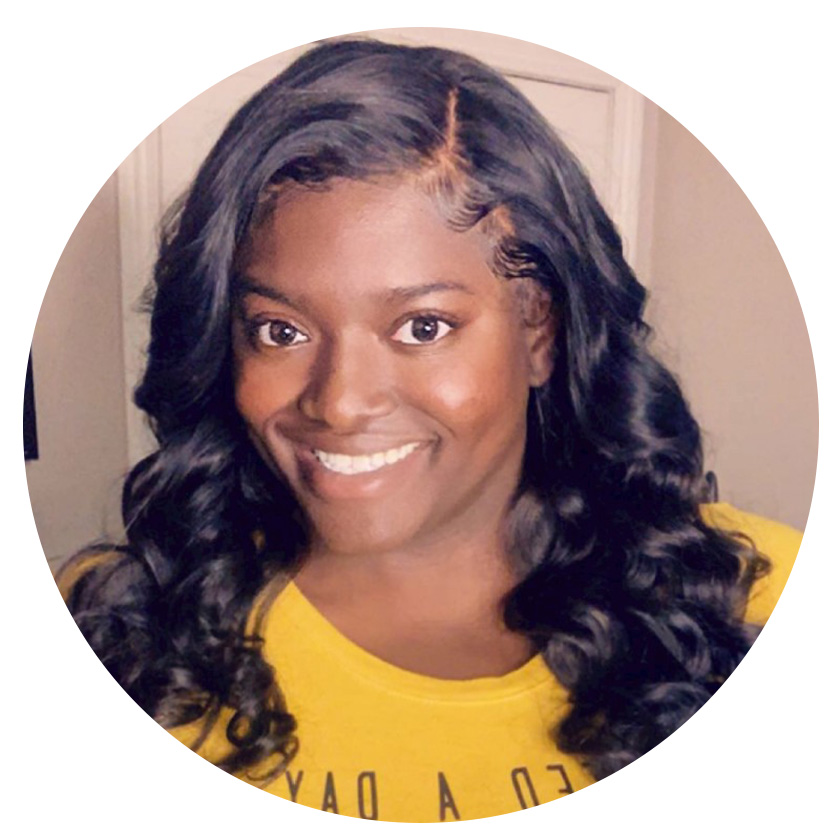
Data Scientist
SambaSafety
One of the issues often debated in AI as it regards to ethics is who is responsible for the social and financial implications of machine learning decisions. I've observed a vast gap between how regulated industries and industries without regulations develop machine learning models.
Credit card companies, risk monitoring, and other consumer reporting agencies care highly about creating models they can stand behind and legally defend, whereas many consumer products don't disclose the nature of their social media research or models.
The same way the insurance and automotive industries consider accidents in a matter of who is at fault, who can we hold responsible when an algorithm predicts poorly?
Some suggest individual engineers should be responsible for the impact their models have on others’ lives. If this were the case we would see a larger emphasis on ethics and data privacy being taught in computer science and data science programs. Are they at fault because it's ultimately their responsibility to choose representative training data and use real data to monitor performance? Data Scientists also can consult with outside companies to audit their data and models.
There are also proponents of policies that would mandate companies that create algorithms that have a drastic impact on human life (in many industries like healthcare and self-driving vehicles it can mean life or death) make their models and training data open to the public. One of the major reasons this is a hard issue to solve is that companies see the algorithms as their “secret sauce”. This business approach has created a high demand and regard for data professionals, however, this comes at the detriment of model transparency.
Many have heard of the Facebook Research project that manipulated users' timelines to measure positive or negative effects. In academic research, there are criteria that proper research must meet to be ethical, especially when the subjects err human. The most egregious offense is the violation of user consent. While buried in the Facebook Terms of Service, few users knew Facebook was using them as emotional test subjects. Internet companies are rarely subject to the stringent criteria of academic research or the explainability of consumer reporting agencies due to few policies that preside over their models.
One of the major issues in determining fault when an algorithm fails is that we don't have comprehensive policies that provide consumer protections and litigation opportunities when we're impacted by a machine learning model. Take the credit card industry for example, in 1970 the Fair Credit Reporting Act was passed to protect consumers from advantageous credit companies. Over the years this has expanded to include many companies who are not credit agencies, but their services can determine whether someone will get a job or not. These companies work to create more interpretable models and prefer using flavors of linear regression over black box modes to provide consumers insight into why a decision was made. Data Scientists in these organizations often prioritize creating models they can defend over black-box models even with state-of-the-art performance.
This brings us back to who is at fault. If an algorithm incorrectly predicts a convicted person will reoffend and in the actual case they didn't reoffend, who owes the convicted person an explanation? Is it the company for having the most resources to rectify the false positive? Do we blame the engineer for not building a more strict model or for including proxies for race and gender? Do we blame the end-user like a county judge for using a tool like COMPASS to predict recidivism without feedback on how well the model works?
We are even having conversations about holding the AI themselves accountable, but that's an intensive task that involves deciding what kinds of consequences or penalties an AI would face after making an incorrect prediction. One solution to simplify the AI responsibility quandary is human in the loop systems. In these scenarios we see AI making recommendations to the human interacting with it. For a remote medical visit, a doctor can receive an image of a growth and have a computer vision program attempt to identify it. The computer can output its
Ultimately, it's up to practitioners, including Data Scientists and Machine Learning Engineers, to follow best practices and mitigate how bias can impact their AI models. To have public access to data models created and used by large firms, we need to enact policies that would incentivize open data. We desperately need a push towards learning AI Ethics in all levels of tech instruction including academia, online MooCs, and bootcamps.
Additional Reading:
Barr, A., 2015. Google Mistakenly Tags Black People as ‘Gorillas,’ Showing Limits of Algorithms [WWW Document]. Wall Str. J. URL https://blogs.wsj.com/digits/2015/07/01/google-mistakenly-tags-black-people-as-gorillas-showing-limits-of-algorithms/ (accessed 1.23.19).
Larson, J., Mattu, S., Kirchner, L., Angwin, J., 2016. How We Analyzed the COMPAS Recidivism Algorithm [WWW Document]. ProPublica. URL https://www.propublica.org/article/how-we-analyzed-the-compas-recidivism-algorithm (accessed 1.23.19).
O’Neil, C., 2016. Weapons of Math Destruction: How Big Data Increases Inequality and Threatens Democracy, 1 edition. ed. Crown, New York.
Recent Posts
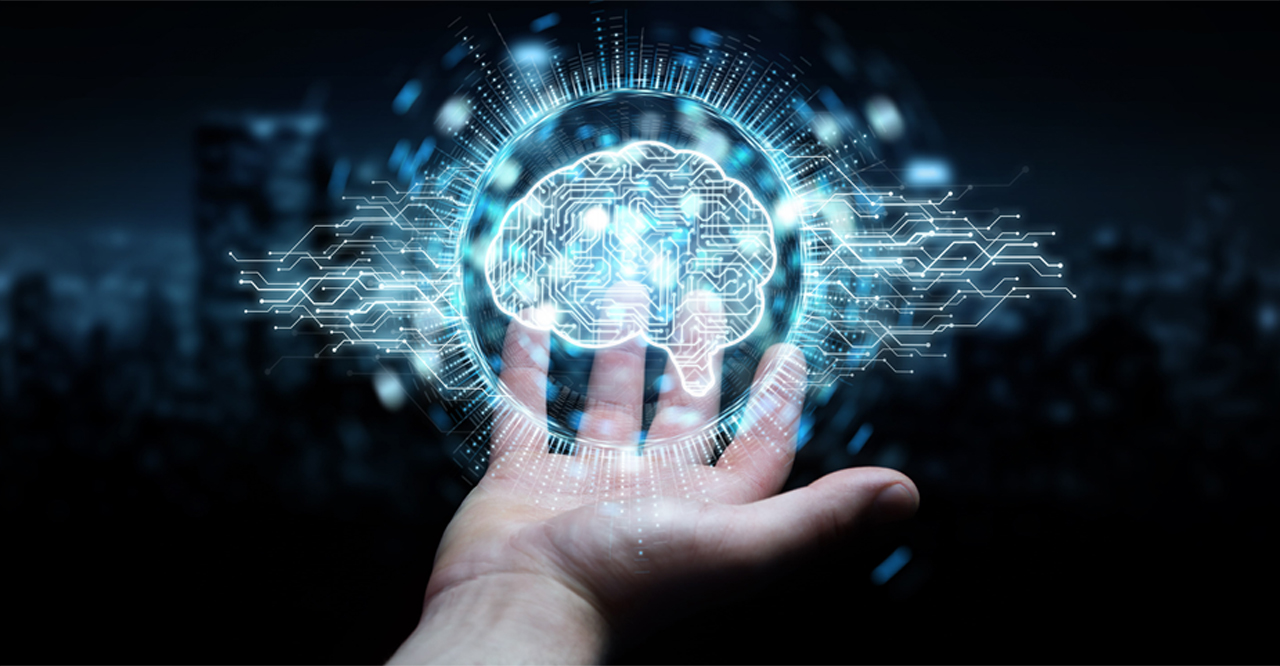
Highlights from 2023: Notable Advancements in AI
As we delve into 2024, it’s an opportune moment to reflect on the dynamic landscape...
By Daniel LacklandApril 26, 2024
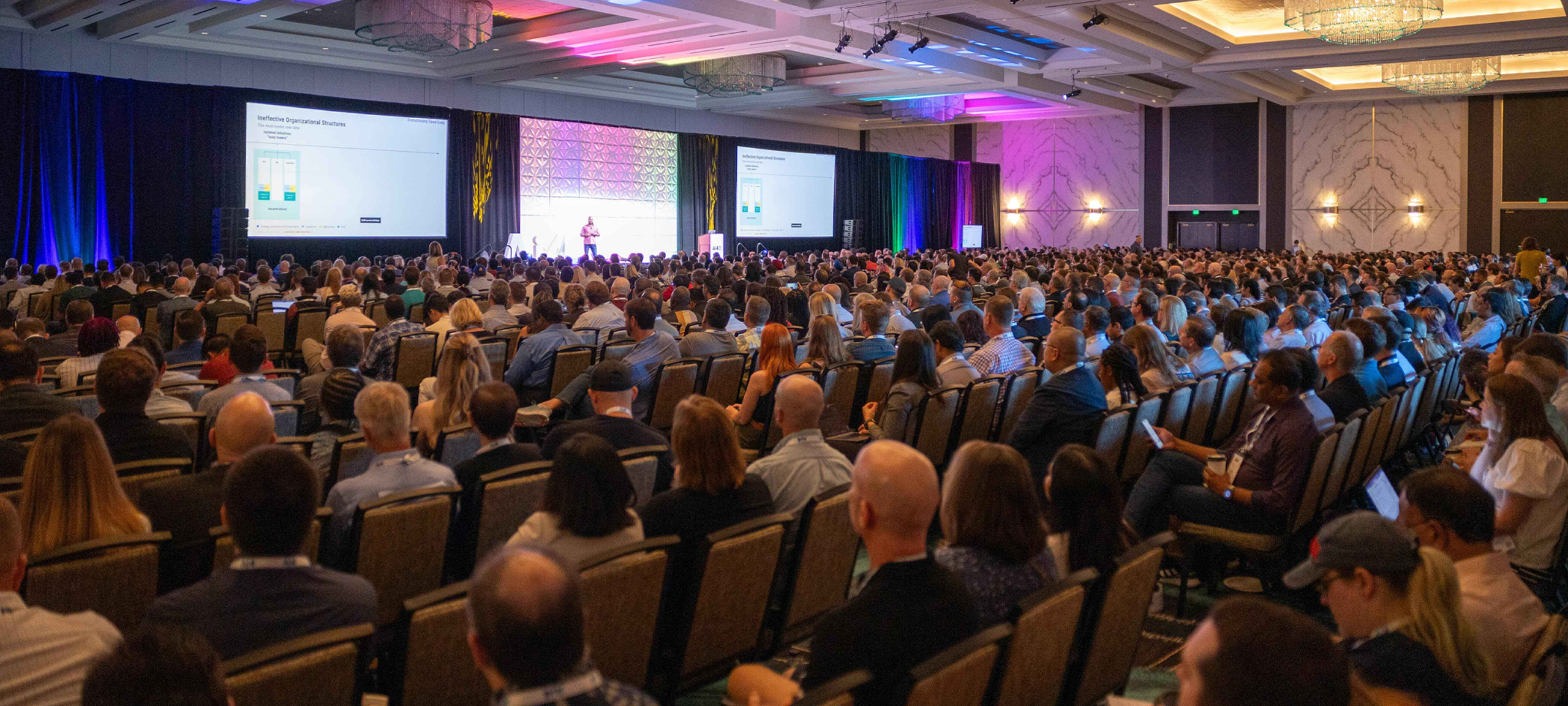
Top AI Conferences of 2024
By Jessica GallagherApril 17, 2024
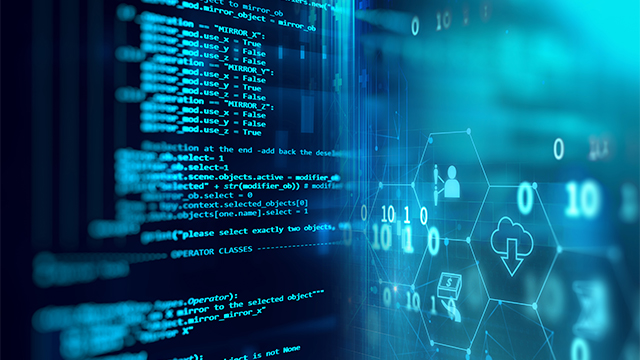
Developing Computer Vision Applications in Data Scarce Environments
Introduction In today’s digital era, computer vision stands as a transformative technology, driving innovations across...
By Sumedh DatarDecember 12, 2023