
During this panel, industry experts (showed above) discussed how hackers are using AI and the changes that they've noticed. We’ve included a short transcription of the panel, beginning at 15:05 of the webinar.
Raffael Marty, Forcepoint: Now that we talk a little bit about what AI is, how have you guys seen that the hackers are applying these methods or have they changed the way they're doing things? Back in the day, they were just attacking any host on the internet through scanning and whatever they find they go and attack it. Are they using artificial intelligence today? And how are you seeing that being used?
Dhia Mahjoub, Cisco Umbrella: I'll jump on the thought I had in the first question then. If we're dealing with AI and adversaries, I can break it into two things: one is how to divert the existing AI to make it harmful. So in a sense, like we said, automation scale and data, for example, you have a lot of open source data nowadays. You can scrape the web, you can download massive scans of the internet, you can download routing tables, you can download all kinds of testing data sets like TLD Zone files and then as an adversary I can go and use algorithms and maybe some machine learning methods to find the vulnerable hosts, let's say WordPress host that is the best for me to attack and leverage for either cyber crime or apt attacks, so that’s one aspect.
Then data collection in mining attackers can leverage that the same way, we leverage it for good and legitimate purposes. My first class of adversary in AI would be the misuse of AI. So examples of that are data mining for harming others. Then there's also surveillance where you have a lot of governments and things like the Snowden controversy and how you had governments spy on their people. You have also governments using some sophisticated methods to go after activists or minorities.
Then there's also misinformation. So again, misinformation has become a lot more and more important and very problematic nowadays from the elections in the US and Europe. So you can use the bot farms, the datasets in the case of Cambridge analytica. That was again, data that people thought was just like harmless and we want to share our likes and friends and now people are leveraging it for malicious purposes.
And then I can also think of automation in malware. So botnets, for example, you can control them remotely and you can give them some sort of intelligence in a sense to kind of group and regroup and then split and change behavior. And so I'll stop there on the first class of misuse of AI.
The second one would be how to attack AI itself. So you have a lot of cybersecurity products, whether it's AV Engine, whether it's DNS-based, proxy based, firewall, and all of them have the AI embedded in the logic to make decisions. Well, you could actually attack them the same way you attack other systems. You could try to find the malware that will be fud, meaning the AI will not detect. You can fud the system to see when it makes mistakes and with the input I gave it worth made mistakes, now I can say the AI system did not recognize this profile, so I'm gonna replicate it and reuse it again to lower it and make it fail.
Raffael Marty, Forcepoint: As seen in recent examples of a very famous endpoint protection company that someone found how to circumvent it by adding a little string at the end of the binaries that came from gaming binary space. That’s a very good point.
Dhia Mahjoub, Cisco Umbrella: Absolutely. The last couple thoughts, for example, let's say I'm thinking specifically about WAF and DDOS mitigation. So those learn from data on the fly. There’s an online perspective or component in there and they tend to be vulnerable because if I keep feeding you some behavior slowly, I'm going to shift your prediction to something I want. It's almost like I'm hiding in the Crowd by making you think that whatever I'm doing now is actually legit even though I'm trying to, let's say, knock some ports. Or I'm trying to, let's say, inject some malicious traffic or SQL injections and stuff like that. So again, to summarize, it's the misuse of AI and it’s also attacking the existing AI that's awesome.
Raffael Marty, Forcepoint: Great. I saw Charles nodding a bunch there when Dhia started talking. Do you want to chime in on some of the things there?
Charles Givre, JP Morgan Chase: Yeah, that was a very thorough response. I was thinking about 4 categories and you really covered it well. Smarter reconnaissance, like if someone is building something to automate and automatically scan networks or IP spaces, they could use their own past experience to build a smarter scanner that can hone in on unidentified vulnerabilities or anything like that, attacking models. This is something that is a very interesting area and recently there were a slew of papers that came out between 2016 and 2018 and I haven't been researching it, but I haven't seen much since then.
But this is a topic that's very interesting to me and I feel like this is something we're going to see as more models get introduced into more and more critical systems. I think we're going to see more and more of those attacks against machine learning models and the example in the paper I saw was that somebody did research and they had a very well trained model that could identify road signs and by strategically putting pieces of tape on the roadside, they were able to get completely erroneous classifications or identification. So you could imagine, if this model was being used in a self-driving car, the implications of something like that. There would be more and more things like that and then of course deep fakes was another category that falls into that.
One of the historical examples of AI being used for attack that I read was about the history of the configure worm. And it used a technique called DGA, or domain generating algorithms. It's a great problem to use to teach machine learning to newcomers. But the essential idea is that the boss gets deployed and they have a random seed and they generate random algorithms that are in sync with the command and control server. So in the first generation of this, these random strings look like just garbage, just gobbledygook. As more and more ML models were deployed to detect this kind of activity, the people developing the botnets came up with new algorithms that used dictionary words. So it became much harder to detect DGAs like that versus the random strings that were generated.
One other thing is that you mentioned that the world seems to have gone through this phase of putting out all this data that was believed to be innocuous. I gave a talk five years ago where I was where I took data that I gathered from the whole bunch of IoT devices in my house. The point of it was to demonstrate how seemingly innocuous data that, when viewed in aggregate, can actually be very very invasive and very revealing about a potential target.
Learn more and watch the full video on YouTube: https://www.youtube.com/watch?v=2ZwxHHHczEA&t
Recent Posts
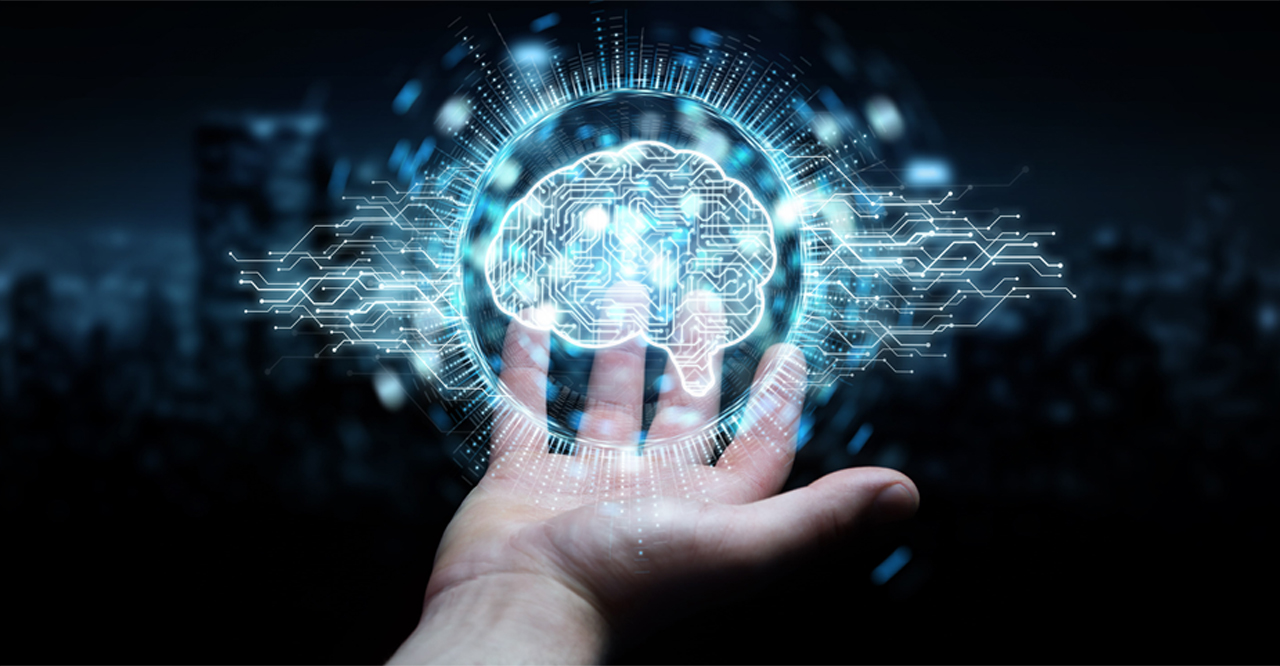
Highlights from 2023: Notable Advancements in AI
As we delve into 2024, it’s an opportune moment to reflect on the dynamic landscape...
By Daniel LacklandApril 26, 2024
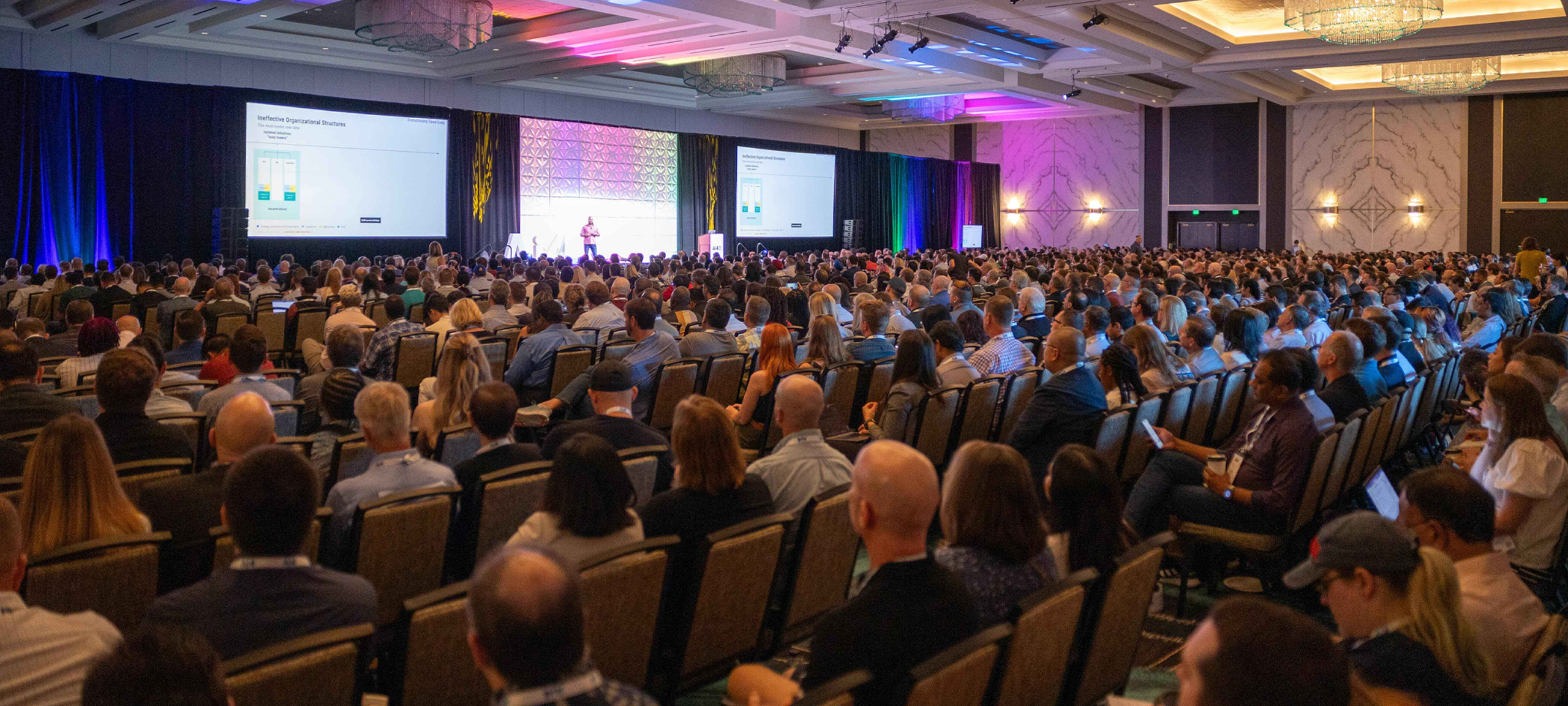
Top AI Conferences of 2024
By Jessica GallagherApril 17, 2024
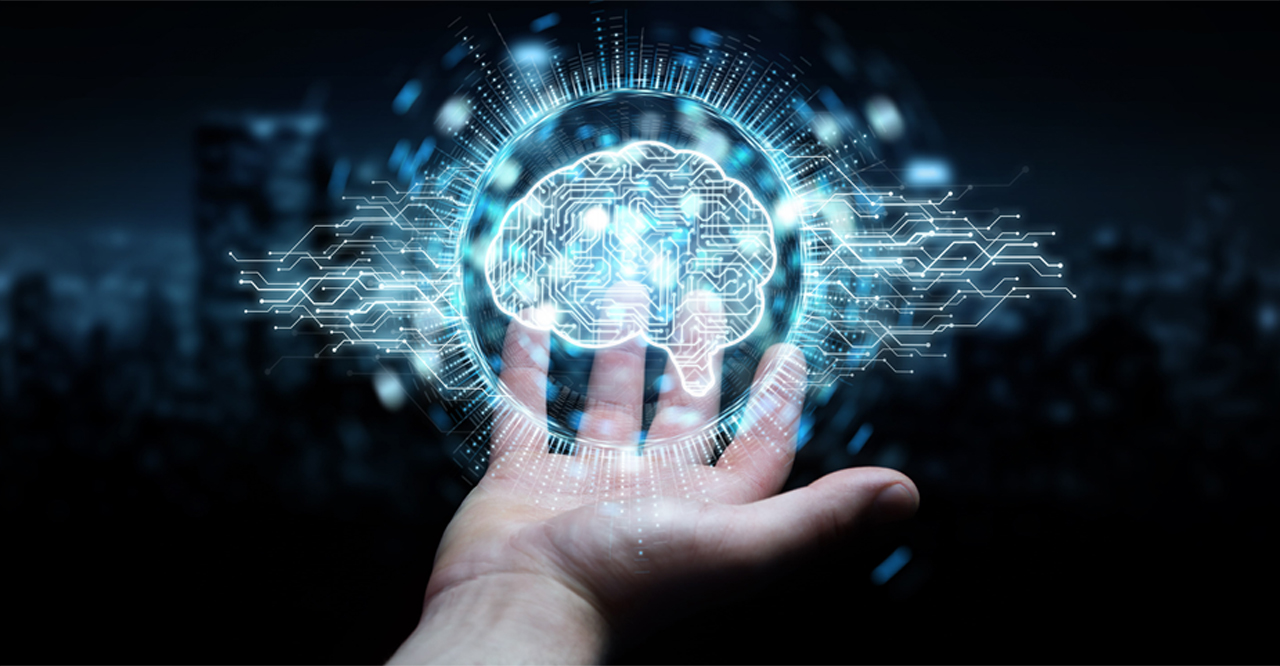
Artificial Intelligence Predictions and Innovations for 2022
Description: Despite artificial intelligence slowing down in 2021, experts believe that 2022 will bring a...
By Emily MooreJanuary 31, 2022