Intelligence is moving to center stage. Traditionally data was shipped away periodically from transactional and customer engagement systems into a faraway data mart or data lake. On these were built business intelligence systems or systems of insights that helped business experts detect trends and plan future course of action. The new normal for business agility makes it necessary to glean insights from data on arrival. Technology now available makes it possible for machines to identify patterns and trends paving the way for intelligence to be available real-time and on- demand.
Insights based on machine intelligence are the new enterprise assets. Organizations are mastering the use of technology to create specific insights. Customers can now receive, for example a personalized product recommendation for wealth creation from within a bank’s app or as a promotion on news aggregator’s channel or while discussing with a financial planner. For sustainable value capture, these insight creation engines need to be protected, monitored, governed, continuously improved, and carefully deprecated at the right time. Insights thus have a lifecycle of their own, automatically making them first class citizens that need to be treated as enterprise assets.
Financial institutions face many challenges in adopting machine intelligence. From a data perspective, sourcing right data, monitoring for any data drift, wrangling data into right form, and understanding context of incoming data are examples. Choice of algorithms, tuning of parameters, explainability of algorithm outcomes, allocation of elastic computing resources for training are some challenges on ML model development. Acceptance of generated insights, its integration into business processes, repeatability of outcomes are challenges while consuming the insights as APIs. Financial institutions who work at intersection of consumers, industry verticals, several ecosystems, legal frameworks, and regulatory directives are far more vulnerable to traps that derail their adoption of machine intelligence.
A playbook approach reduces or eliminates getting stuck in traps. It guides stakeholders to a fair amount of success in their transformation journey. In the recent past, when APIs were identified as assets, their adoption was promoted through structured approach, identifying different use cases, encapsulating system use cases and championing design approaches. Taking a leaf from that movement, we propose creating an insights layer to nurture insight assets. It needs to be specific to each organization, customized for their business context and designed for safety, resiliency, and sustainability.
Figure 1 shows a holistic view on how organizations can perceive the transformation. For insights to become machine driven and available on-demand, organizations need to move to a mindset of insights layer from existing system of insights approach. Being a logical layer, existing system of insights can coexist, evolve, and gradually become part of insights layer.
We have articulated several components of the insights layer earlier(1). While advising customers, we categorize implementation from two perspectives – learning (derive insights) and deployment automation (consume insights). Our interactions with several financial services organizations across the globe is helping us understand real issues that are preventing widespread AI adoption. With insights layer, we believe we can tie many loose ends together, helping better and faster adoption of machine intelligence.
Should every enterprise focus on developing an insights layer and redirect scarce resources from their data science programs? While quantity of insights is a good measure, we advise a guiding mechanism that is based on scope of business value delivered. Another measure that is complementary as well as orthogonal is the need for keeping the insights current and available.
Figure 2 is a typical 3-zone square, that guides executives in determining investment for insights layer. On the horizontal axis is coverage of insights. Localized implementation does not need an elaborate insights layer. However, when multiple capabilities depend on an insight asset, focus on its usage, monitoring, control, and governance is necessary.
On the vertical axis is the stability of insights. Outcome from an insight asset change if input data profile change, for example. They need to be continuously monitored and need finetuning. This calls for a cadence and a focus on automation within the insights layer paradigm. However, a one-off insight asset that is created, for example during a specific marketing campaign, it might not need an investment in a structured approach.
In this article, we have introduced an idea for creating an insights layer and a guideline for adopting one. Through our work with customers, we will flush out more details on the key construct and share details in future.
References:
(1) Our earlier paper on SAIL framework to achieve the vision of insights layer:
https://www.americanbanker.com/whitepaper/insights-at-scale-new-growth-engine-for-financial-institutions
Recent Posts
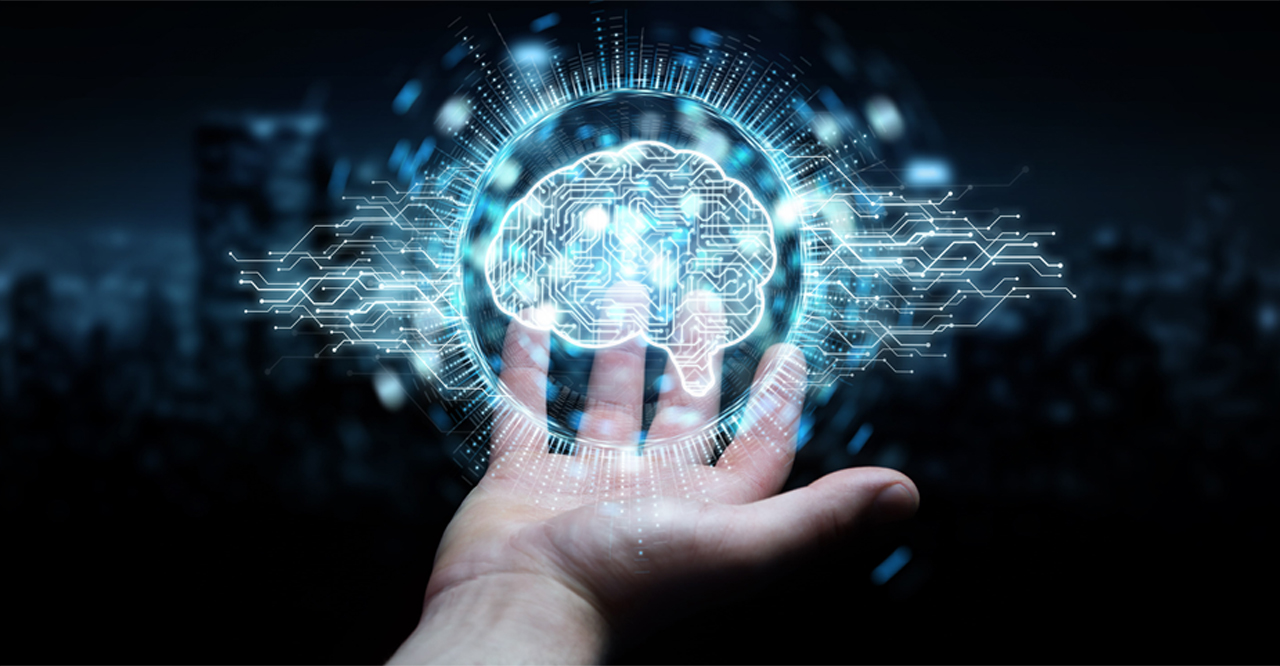
Highlights from 2023: Notable Advancements in AI
As we delve into 2024, it’s an opportune moment to reflect on the dynamic landscape...
By Daniel LacklandApril 26, 2024
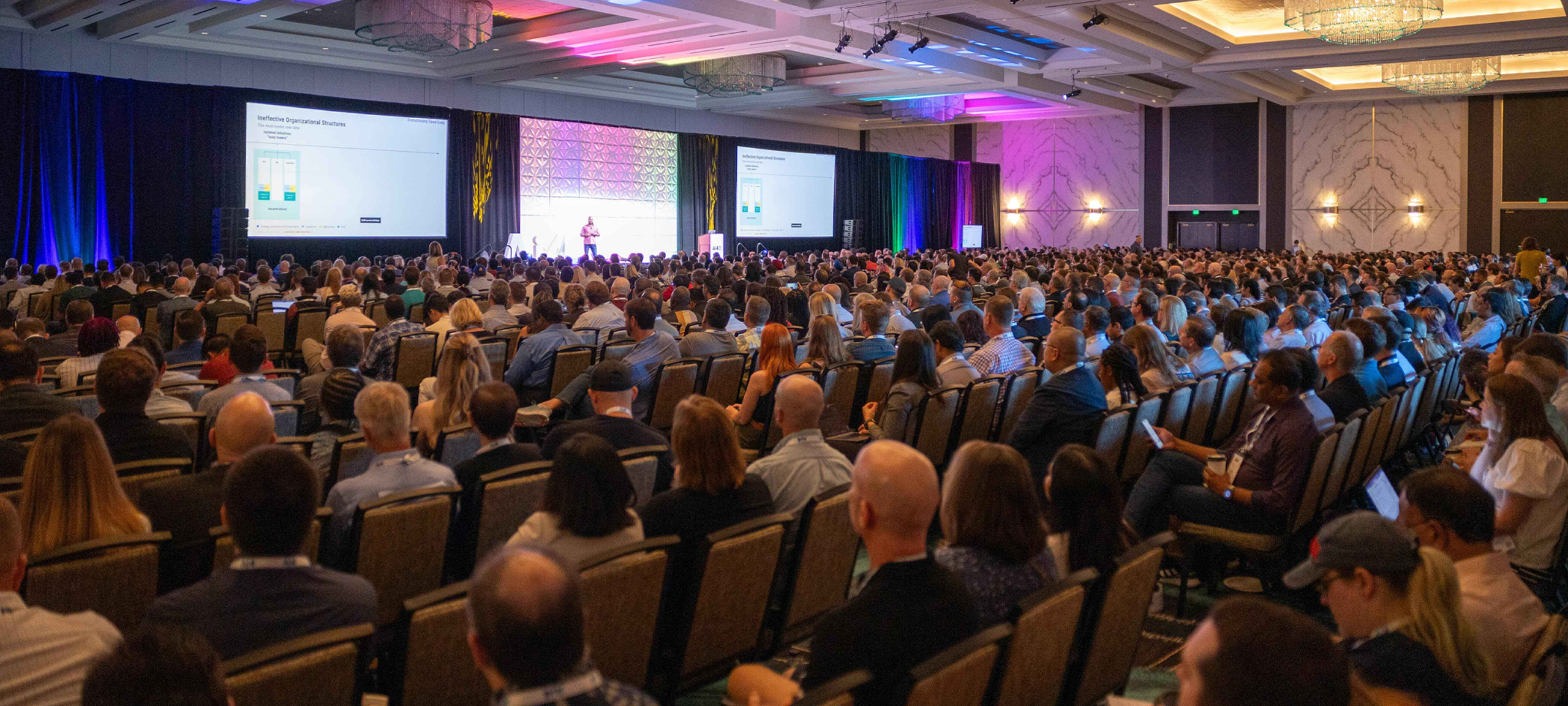
Top AI Conferences of 2024
By Jessica GallagherApril 17, 2024
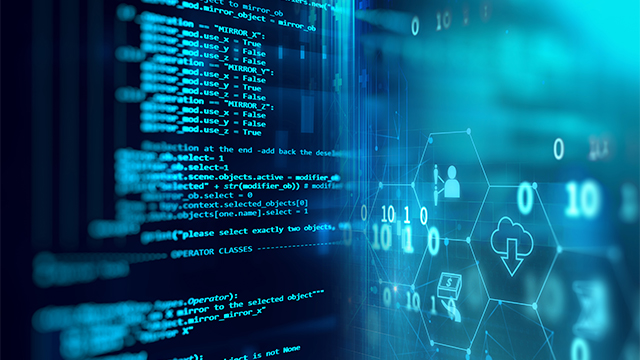
Developing Computer Vision Applications in Data Scarce Environments
Introduction In today’s digital era, computer vision stands as a transformative technology, driving innovations across...
By Sumedh DatarDecember 12, 2023