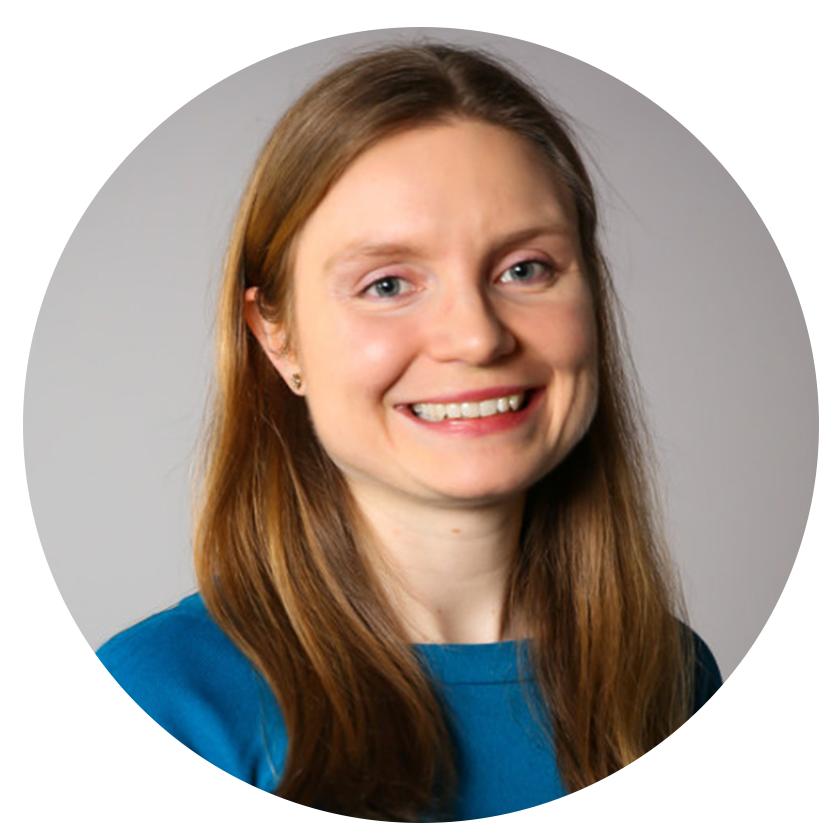
Senior Data Scientist
Macy's
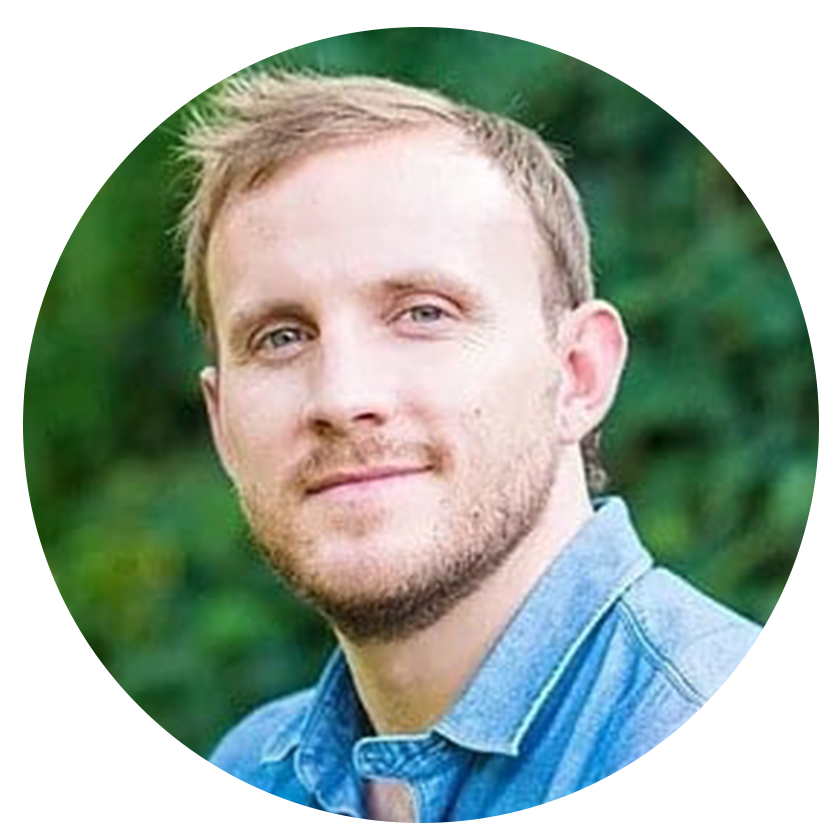
Data Scientist
Macy's
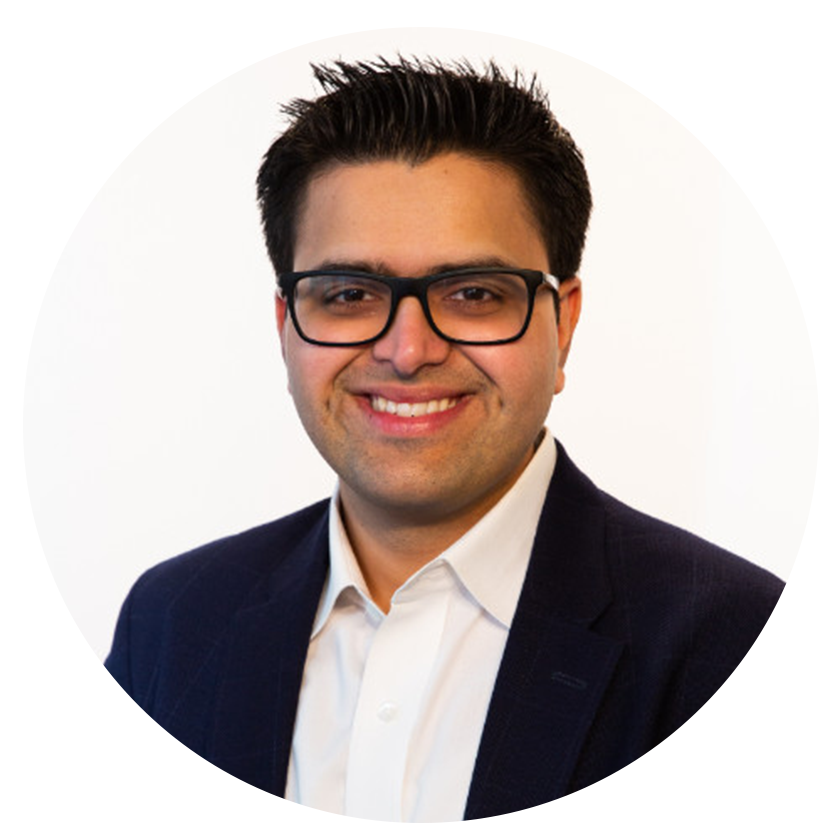
VP, Data Science
Macy's
Overview
In this presentation a team of Data Scientists, Bhagyesh Phanse (VP), Jolen Mork (Senior DS), and Iain Stitt (DS), from Macy’s discuss the challenges and solutions reached in product forecasting in the retail market. The Macy’s team discusses two different issues they are facing determining the automation of markdown decisions for products as well as using unsupervised machine learning algorithms to cluster stores together based on seasonal sales patterns.
Bhagyesh begins this presentation speaking to how machine learning is applied across Macy’s as a company. With the retail space changing quickly, there are opportunities to apply machine learning to almost every aspect including marketing, advertising, pricing, and supply chain management. The question becomes where should the resources for machine learning be allocated? The specific problems of what is trying to be solved must first be determined followed by an evaluation of how big of an impact could potentially follow from implementing machine learning algorithms in each respective area. By having a large scope of what machine learning can possibly be applied to allows for and also requires cooperation across the board for proper implementation.
“By investing in analytical and computing power, it allows us to explore new areas of application from a collaborative perspective.”
Next, Jolen talks about when, where, and how much a product should be marked down. In the Macy’s retail market space, a unique problem is presented in trying to predict the trends for fashion products that have never been sold before and will never be sold again. Questions such as what will the demand be and where should distribution be focused from a geographical and online perspective are a few of the questions that need to be answered.
In order to try and minimize liquidation of products, Macy’s attempts to use markdown pricing to optimize sales by manipulating the prices. Being able to get business feedback is crucial to be able to update the machine learning algorithms to try and best understand how consumers react to a specific product. This kind of demand forecasting, where a comparison between sales trends and price reductions is made, is a common reinforced learning method used throughout machine learning algorithms.
Iain then goes on to speak about how different stores are grouped together using unsupervised machine learning techniques. Where Jolene speaks to how individual product demand can be predicted, Iain speaks to how trends experienced amongst several related stores can give more accurate predictions given the correct conditions. When determining the seasonal demand of a product, being able to look at seasonal rates alongside seasonal averages can be an effective way to determine the underlying rate function from observed sales points. As is typical with most machine learning techniques, the more data you have the better.
Being able to group stores together allows for more data to be used and more accurate predictions be made. Using a technique called spectral clustering, data is grouped together using a K nearest neighbor modeling technique. Stores are grouped together based on similarities of seasonal sales patterns. Distance formulas are then applied to determine the closest ‘node’ each data point within a graph below to. The number of nodes is typically an arbitrary number that is chosen that shows the total number of groups that the model wants to display.
Macy’s have invested heavily in applying machine learning algorithms and models to their everyday business. This has allowed them to optimize product placement geographically and product pricing within stores. It has allowed them to accurately update pricing based on demand forecasting. Company’s today continue to find new ways to optimize their business from a statistical perspective. It is a different way to look at common problems and remove typically biases that would normally result in different outcomes.